By decoupling prediction from human judgement, AI promises to turn organisations upside down, argue three Canadian academics in an acclaimed book. Alex Katsomitros finds out more
When the covid pandemic started in 2020, University of Toronto academics Ajay Agrawal, Avi Goldfarb and Joshua Gans were confronted, like everyone else, with a big problem: staying away from the virus. Our lack of information meant that a single case at work could leave hundreds of uninfected people at home. Along with other experts, they developed a rapid antigen testing program to predict who had been infected, effectively turning a health problem into a prediction one. As they would soon find out, rigid rules governing several aspects of the social distancing system, from privacy to data security and waste disposal, did not facilitate information-based decision-making. After deliberations with various luminaries, from former Bank of England governor Mark Carney to author Margaret Atwood, they partnered with twelve Canadian firms to remove barriers that blocked the implementation of their program. Eventually, it was adopted by more than 2,000 organisations, helping them to keep the wheels turning.
Decoupling prediction from judgement
Moving from catch-all rules into customised decisions will soon be necessary due to the rise of AI, Agrawal, Goldfarb, and Gans argue in their new book, Power and Prediction: The Disruptive Economics of Artificial Intelligence. That AI is a disruptive force is a common theme of many books, including one that the academic trio have written; that it takes systematic organisational change to tap into its full potential is a more provocative argument. In their previous book, Prediction Machines, the authors claimed that advances in AI are essentially an improvement in prediction technology, a view embraced by various leading names at Silicon Valley. But as the authors acknowledge, they had underestimated the economics of building AI-driven systems. “We had focused on the supply side and we underappreciated demand. The only firms that quickly adopted AI were those where you could adopt it as ‘a point solution’, and keep the rest of the system the same,” says Agrawal from his office in Toronto, adding: “Anything that requires system-level change is harder. You need to change other factors, including job descriptions, information flows, the way work is performed, incentive systems.”
To show the challenges involved, the authors delve into the history of innovation. When electricity first appeared, enthusiasm for its potential did not translate into immediate benefits, as it was initially seen as a cheaper steam engine. It would take entrepreneurs four decades to realise that its value lay in decoupling energy use from its source, freeing consumers from the constraint of distance and enabling more efficient factory design. As with the early stages of electricity adoption, AI is currently in what the authors call “The Between Times”; although it has demonstrated its potential, its applications remain mere improvements to existing systems. Indeed, it follows that AI has only had a meagre impact on economic growth and productivity rates so far.
AI’s value added, the authors argue, lies in decoupling prediction from the rest of the decision-making process, notably human judgement, that can facilitate more productive organisational design. One example is car insurance. AI assistants attached to cars can predict the likelihood of an accident, while customers use their judgement to calibrate actions, with premiums determined by the possibility of an accident and potential repair costs. Tesla already uses vehicle data to measure safety scores based on driving behaviour. “Judgement is knowing which predictions to make and what to do with those predictions when you have them,” says Avi Goldfarb. “It represents the decision to deploy an AI and whether to automate a process or put a human in the loop.” Many psychologists would argue that judgement will still be shaped by the perceptions and biases through which we interpret AI-generated predictions, rendering this separation impossible. “When we decouple prediction from judgement, AI forces us to have greater clarity around our decisions in terms of what our objectives are,” Agrawal counters. “Right now, we can have muddled objectives, because we don’t have to distinguish between our prediction and judgement. AI requires explicit decisions about what we value.”
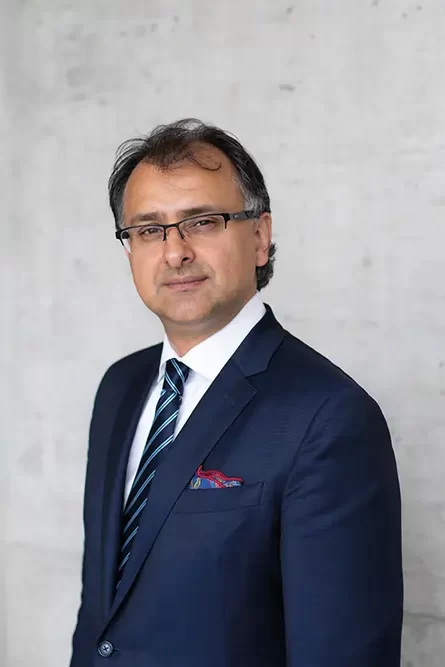
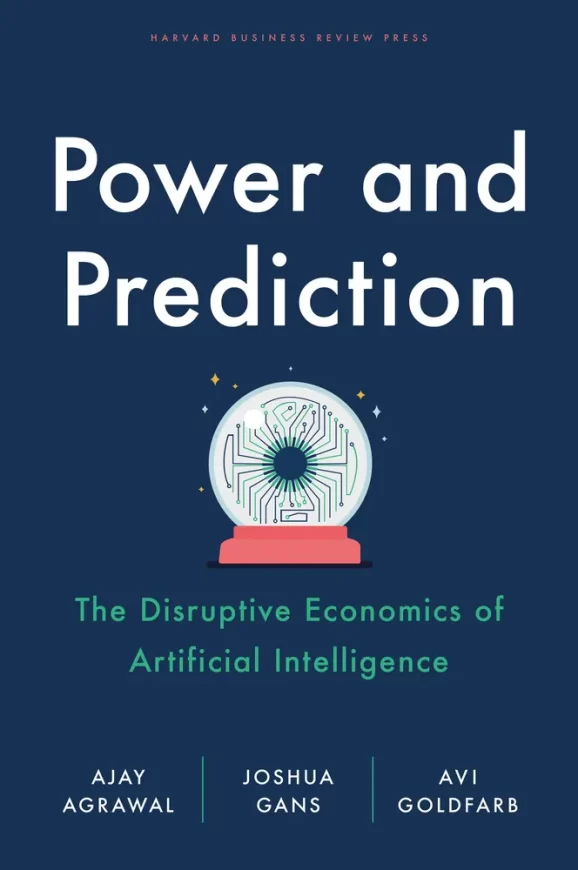
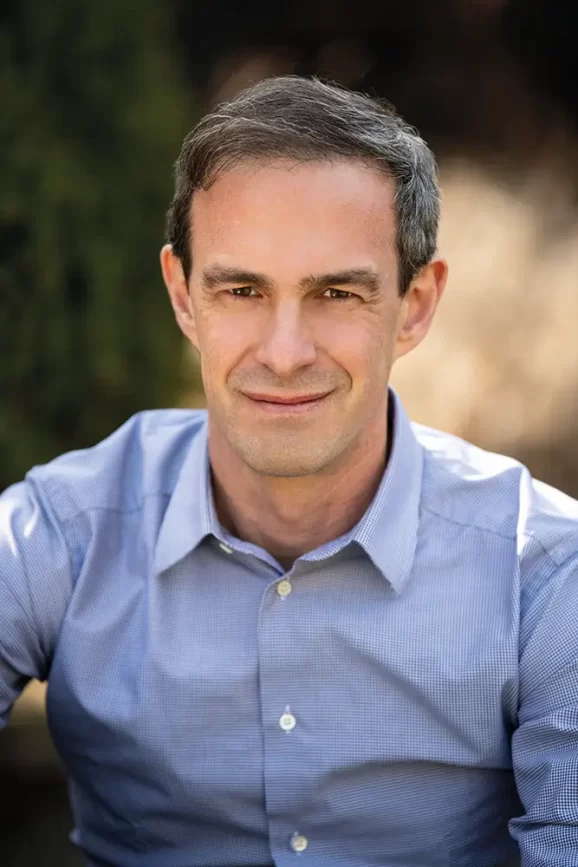
Data-driven monopolies
Like other tech niches, AI is a market vulnerable to winner-takes-all monopolies. The more data AI tools are fed with, the better predictions they can make, conferring an advantage on first movers. It’s no coincidence that many Silicon Valley powerhouses have rushed to enter the race. In the case of self-driving cars, “Nobody’s gonna want to buy a car that’s driven by an AI and is substantially worse than another one,” the authors argue. “Dominance by a small number of companies in AI might limit the direction of innovation to areas that favour existing technology company strategies,” says Goldfarb, adding: “We need vigorous antitrust enforcement of existing laws to ensure that companies are unable to leverage current market power to dominate future AI markets”. What about calls by Elon Musk and other tech leaders for a pause on AI research and deployment? One solution would be to set up registries, Agrawal suggests, so that governments know who is building what, even if the possibility of non-compliant rogue actors getting an advantage remains strong. However, he fears that restrictions may make things worse. “It will slow down potential progress. There are already people dying today because of friction that has slowed down implementation of AI in the healthcare system,” he says. “AI substantially reduces the cost of diagnosis for many lower-income people and restrictions are preventing that.”
For organisations deploying AI, the necessary organisational changes will be enormous and divisive, given that those losing power will have a vested interest in maintaining the status quo. As the authors note, machines may not have power themselves, but they can reallocate it to those who can exert better judgement by changing the time and place decisions are made. Resistance may come from a wide range of stakeholders, from employees to suppliers. Despite its early experimentation with on-demand video, Blockbuster, the dominant US videotape rental chain store in the 1990s, lost out to Netflix and eventually folded out of fear of antagonising owners of franchise stores. “CEOs still struggle, because incentive systems, how people earn their bonuses and how they get promoted, are based on systems set up for the way their business works today. It’s like an immune system that rejects an organ transplant,” Agrawal says. “It takes an incredibly visionary leadership at a large company to implement a big change like that. In most cases, it’s a new entrant who does it.”
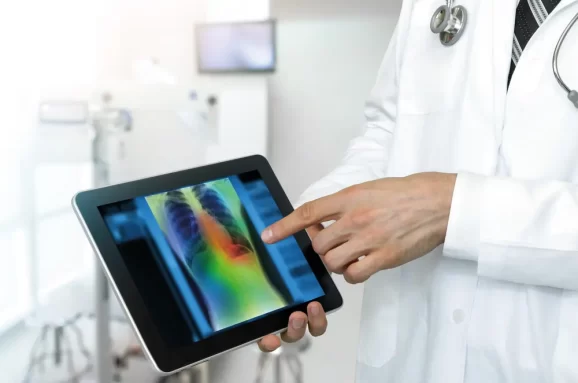
Towards a new education model
One area where AI is already changing the landscape is education. The authors use the medical profession to show how dramatic things may soon become. Medical schools, they argue, may no longer require memorisation of facts, or select students based on biology testing performance, while physicians’ current patient-facing experience might become irrelevant. Should we be worried that we might lose crucial skills? Agrawal turns to accounting to show the absurdity of the question. In previous decades, he explains, accountants were adding up all the phone numbers from phonebook pages to practise adding and subtracting, until calculators and spreadsheets rendered those skills obsolete. “Today, we still have many accountants, but nobody does adding in their head anymore,” Agrawal says. “Accountants use their judgement about what to do with the numbers they produce and somehow nobody is concerned that they are losing the brain power of how to do addition. Down the road, we will feel upset if somebody tries making similar predictions in their head.”
Alarmists raise the prospect of AI-driven massive unemployment. The question of whether machines are now the bourgeoisie to our proletariat is absurd, the authors argue, given that decisions still come from people. “It’s time to stop worrying that robots will take our jobs,” they write, “and start worrying that they will decide who gets jobs.” One example is the London taxi industry. Traditionally, taxi drivers go through a rigid three-year training period, memorising street names to identify the fastest routes. When AI-driven apps came along to generate those predictions, nothing changed for existing taxi drivers, but AI enhanced the value of judgement of Uber drivers, expanding the set of workers who could enter the profession.
So what skills will keep people employed forever? Surprisingly enough, Agrawal suggests a return to a more traditional education model. “We have over-indexed STEM subjects and undervalued the arts and the humanities, which is where we learn judgement. Judgement is learning how to make trade-offs,” he says, adding: “Machines have zero capability of judgement. We’re taking a risk by underinvesting in the humanities, because that is where our values are passed from generation to generation.”